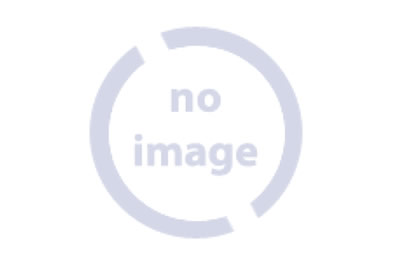
May
KIU Rangers Ease Past UPDF Lady Tomahawks
May 3, 2024, 4:46 pm
Title: | Revolutionizing Coffee Farming: A Mobile App with GPS-Enabled Reporting for Rapid and Accurate On-Site Detection of Coffee Leaf Diseases Using Integrated Deep Learning |
Author(s): | Eric Hitimana 1,* , Martin Kuradusenge 1 , Omar Janvier Sinayobye 1 , Chrysostome Ufitinema 2, Jane Mukamugema 2, Theoneste Murangira 3, Emmanuel Masabo 1, Peter Rwibasira 2 , Diane Aimee Ingabire 1, Simplice Niyonzima 1, Gaurav Bajpai 4, Simon Martin Mvuyekure 5 and Jackson Ngabonziza 6 |
Year | 2024 |
Publisher: | MDPI Software |
URI: | https://kiu.ac.ug/publication-page.php?i=revolutionizing-coffee-farming-a-mobile-app-with-gps-enabled-reporting-for-rapid-and-accurate-on-site-detection-of-coffee-leaf-diseases-using-integrated-deep-learning |
File: | |
Keywords: |
Coffee leaf diseases are a significant challenge for coffee cultivation. They can reduce yields,
impact bean quality, and necessitate costly disease management efforts. Manual monitoring is laborintensive
and time-consuming. This research introduces a pioneering mobile application equipped
with global positioning system (GPS)-enabled reporting capabilities for on-site coffee leaf disease
detection. The application integrates advanced deep learning (DL) techniques to empower farmers
and agronomists with a rapid and accurate tool for identifying and managing coffee plant health.
Leveraging the ubiquity of mobile devices, the app enables users to capture high-resolution images of
coffee leaves directly in the field. These images are then processed in real-time using a pre-trained DL
model optimized for efficient disease classification. Five models, Xception, ResNet50, Inception-v3,
VGG16, and DenseNet, were experimented with on the dataset. All models showed promising
performance; however, DenseNet proved to have high scores on all four-leaf classes with a training
accuracy of 99.57%. The inclusion of GPS functionality allows precise geotagging of each captured
image, providing valuable location-specific information. Through extensive experimentation and
validation, the app demonstrates impressive accuracy rates in disease classification. The results
indicate the potential of this technology to revolutionize coffee farming practices, leading to improved
crop yield and overall plant health.
Kampala International University,
Box 20000, Ggaba Road, Kansanga, Kampala